LGO interns interested in making something new can do an internship in product design and development. Projects in this category allow students to combine their engineering and MBA knowledge in a unique way to solve problems related to design, product implementation, and new supply chain systems.
Prototyping of Injection EVA Foam Footwear Midsoles
Amit Galgali (LGO ’22)
Engineering Department: Mechanical Engineering
Company: Nike
Location: Beaverton, OR
Problem: Nike’s Product Creation Center (PCC) is a facility where shoe prototypes are developed and fabricated. However, the PCC does not have a complete capability to make midsoles out of injection-EVA foam, also known as injection-phylon (IP), instead outsourcing them to factories across Asia. The goal of Amit’s internship project was to develop a plan to introduce a supplementary IP midsole capability at the PCC. This would increase overall prototyping capacity and enable the PCC to undertake more experimental projects poorly suited for outsourcing, potentially reducing development time and increasing innovation.
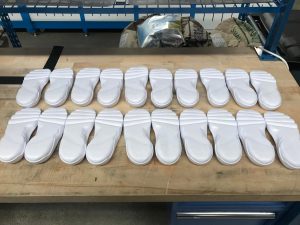
Approach: To achieve this, Amit first gathered domain knowledge from literature, conversed with experts at the PCC, and shadowed current PCC processes. He then conducted a trial with a top Asian factory to remotely benchmark the factory, understand their institutional knowledge, and then test the PCC to determine best practices and gaps in three key stages of the IP process (ER grading, mold design, part making). This was done by designing and fabricating a chosen IP midsole in both locations and then comparing physical samples.
Impact: The PCC was able to develop 3 sets of the chosen midsole; each set designed to isolate and assess a different stage of the IP process. Testing and analysis of the parts compared with those from the Asian factory concluded the PCC could successfully produce IP midsoles that met almost all specifications using existing equipment and knowledge transferred from the Asian factory. The trial highlighted areas of further work to be conducted as part of a roadmap that would enable the PCC to implement a complete IP midsole capability.
Implementing Large Format Additive Manufacturing in Aerospace Tooling
Phil Cotter (LGO ’21)
Engineering Department: Aeronautics & Astronautics
Company: American Industrial Partners (Ascent Aerospace)
Problem: Ascent Aerospace designs and manufactures a diverse array of customized aerospace tooling, creating a low-volume/high-mix production environment where precision is critical. Additive Manufacturing (AM) can provide a significant competitive advantage by reducing lead time, cutting costs, and enabling the rapid production of novel tooling solutions. Ascent recently installed a Large-Scale Additive Manufacturing Machine (LSAM) to exploit this opportunity. Phil’s project explored the deployment of this new technology via process integration and finite element analysis (FEA) of print performance.
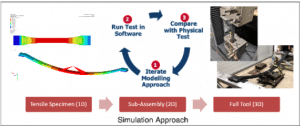
Approach: Phil developed an understanding of the current state of traditional manufacturing processes at Ascent and how the LSAM’s capabilities could be integrated in a future-state process. To improve design cycle time, reduce rework, and improve quality, Phil developed an FEA model of LSAM-printed tools. Comparison between the FEA model and the results of physical tests were then built into the framework for LSAM integration.
Impact: Phil’s project created a test plan and operational improvement initiatives to establish the LSAM’s functionality, reduce variability in its operation, and capture best practices. His methodology for simulating and testing the performance of LSAM-printed objects paved the way for onboarding new feedstocks and developing novel tooling designs. Beyond Ascent’s specific context, many of the processes and approaches developed for this project can be applied by other manufacturing operations to efficiently integrate large format additive manufacturing.
Drug Substance and Drug Product Manufacturing Strategy Assessment for siRNAs
Monica Gabriela (LGO ’21)
Engineering Department: Civil and Environmental Engineering
Company: Amgen
Problem: Amgen has multiple Small Interfering RNA (siRNA) programs in development, with the first asset expected to be released to market soon. Amgen traditionally outsourced the drug substance and drug product manufacturing of siRNAs. But with the growing siRNA portfolio, Amgen must decide if they should continue to outsource the production or start to manufacture in-house, either using existing facilities or building new sites.
Approach: Using information from demand projections, production data and efficacy, new facility cost approximation, capacity planning and progression data, and operational data, Monica created a supply chain model to analyze the resilience and weak links of Amgen’s siRNA supply chain. The model produced a risk-to-cost input for an overall business model – equipped with mixed integer programs – which can help to identify the scenario with the least 20-year present value of expense.
Impact: Analysis revealed that there is a potential loss from internalization in year-0 of up to $13 million if one of the most sensitive parameters, the demand level, is low. Although the analysis suggested that Amgen revisit the study in year-13 or year-14 – when the demand for its first siRNA asset is more developed – Amgen will regularly update and analyze the business model based on the most up-to-date input numbers.
Leveraging Flexible Manufacturing to Streamline New Product Introduction Processes
Taylor Robinson (LGO ’20)
Engineering Department: Mechanical Engineering
Company: Johnson & Johnson
Location: Jacksonville, FL
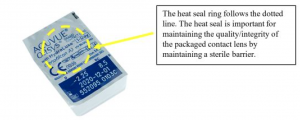
Problem: Johnson & Johnson Vision Care (JJV) is committed to launching new ACUVUE® Contact Lens products yearly, but current manufacturing lines operate at high utilization rates to meet growing demand. This limits the opportunity to test and validate new lenses for commercial-scale production. Using the JJV Flexible Manufacturing Platform (FMP), which applies innovative and flexible manufacturing technologies, Taylor built a case study to explore strategically leveraging FMP to enable quicker transitions from pilot-line to commercial-scale production.
Approach: Taylor’s FMP case study focused on the heat seal manufacturing step, which was chosen due to its critical importance in maintaining a sterile barrier. The case study also provided further evaluation of the overall manufacturing line, which previously lacked consistency and reliability due to obstacles arising with integrating a new technology. Testing to measure the impacts of contact time, temperature and pressure, the case study showed that time and temperature have the highest influence on heat seal integrity.
Impact: Results from the heat seal case study were used to create a process capability model that ultimately improved decision quality and reduced product failures by 80%. In the long term, the FMP evaluation showed that there is capacity to launch new contact lens products yearly while creating less waste during the product design and development stage. FMP can become a strategic and efficient asset to continuously launch new products for companies such as JJV that have high-volume, high-mix product lines.
Process Enablers for Successful Reverse Engineering inside Large Organizations
Casey Boyle (LGO ’20)
Engineering Department: Mechanical Engineering
Company: Boeing
Location: Renton, WA
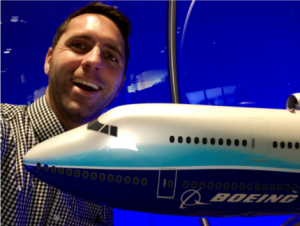
Problem: Reverse engineering can be a productive and strategically advantageous process for large companies to stay competitive and improve their business and expertise. Yet only a small percentage of reverse engineering projects actually reach completion. Casey’s project sought to understand and outline the characteristics of an effective reverse engineering workflow and organization.
Approach: Casey identified key enablers to successfully promote reverse engineering at scale through literature review, assessments of Boeing’s teardown process, and overall component design flow. He determined that reverse engineering should be a system of interconnected dependent events to build a standard workflow around the teardown process guided by functional analysis and clear communication among stakeholders.
Impact: The “pull-” more than “push-” focused process Casey built establishes clear communication between functions and can prevent rework, shorten flow time, and increase quality. He created a framework for implementing these recommendations and tools to mitigate project risks, increase the efficiency of reverse engineering teams, and reduce the occurrences of downstream rework. While no single enabler is in itself novel, when aggregated into a system of best practices, the organization becomes more capable.
Picking Winners: Predictive Modeling for Cell Line Selection
Yucen Xie (LGO ’19)
Engineering Department: Chemical Engineering
Company: Amgen
Location: Thousand Oaks, CA
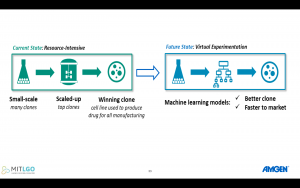
Problem: In the discovery-to-market development cycle for biopharmaceuticals, the manual selection of a cell line for the Master Cell Bank in product manufacturing for clinical and commercial use is a time-consuming, complex, and resource-intensive process. The selection process is largely conducted on a per-experiment basis to select a single cell line that can yield drug products of consistently high quality and titers.
Approach: Yucen’s project aggregated pre-clinical data to create analytic tools utilizing machine learning algorithms, to produce insights and predictions for cell line selection. He built and implemented an integrated modular virtual experimentation package through data extraction, feature engineering, model evaluation, and user interface design to reduce prediction errors by 38% to 90% for bioreactor end-point titer and product quality metrics.
Impact: Yucen’s models generated parallel in silico predictions with high accuracy, leading to better knowledge of key attributes affecting titer and product outcome, more productive cell lines of higher quality, and reduced development cycle times. This modular algorithmic framework and novel application of machine learning also promotes a scalable and transferable digital platform for analogous applications within the biopharmaceutical industry. Amgen has since submitted a patent application as a result of Yucen’s work.